A/B testing is a methodology to test marketing tactics, with the objective of optimizing results over time.
In this article, I’ll explain my approach to A/B testing in Google Ads, with a focus on search and PMax campaigns.
What Is A/B Testing in Google Ads?
Generally speaking, A/B testing, also known as split testing, is when marketers compare a baseline version of a marketing tactic (A) to a test version, ideally with a single difference (B). It is considered an essential approach to SEM optimization.
In order to get clear results, marketers should only change one element between their baseline/control version and the test version.
If you test different ad copies, given the nature of RSAs, I usually change a significant number of the headlines and descriptions. In most cases, the test is comparing between ‘angles’, or ‘styles’, not the specific headlines and descriptions.
Here are a few examples from Google Ads:
- Campaign bid strategy: Compare tCPA to tROAS
- Ad variations: Compare an ad that focuses on a product’s good value for money vs. its quality
- Landing page: Compare an ad that sends users to a product page vs. a listing page
- Audience signals (PMax): Test an asset group with open targeting (i.e. no audience signals) to another with remarketing and in-market audiences
For each test, it’s important to define its objective – why we run this test; a hypothesis – what results we expect to see at the end of the test; and the criteria (metric) on which we’ll define the winning version.
The duration of each test will depend on the amount of data in your campaign. The more data you have, the faster you’ll be able to see significant results.
Coming back to the examples mentioned above, it would look something like this:
A/B Test | Objective | Hypothesis | Criteria (metric) |
Campaign bid strategy | Increase conversions | tCPA would get more conversions at a similar CPA | Conversions increase |
Ad variations | Increase CTR | Ad copy that focuses on value for money will have a higher CTR | CTR increases |
Landing page | Increase CVR | Product pages will have a higher CVR vs. listing pages | CVR increases |
Audience signals | Decrease CPA | Asset group with signals will have a lower CPA | CPA decreases |
What Are The Different Types of Testing in Google Ads?
There are different ways to set up A/B tests, depending on what you’d like to test.
Here’s a rough overview of approaches to A/B testing in Google Ads:
- Experiments: For split testing (when the campaign has enough data)
- Time-based: Run version A, pause it, and run version B (when the campaign has little data)
- Geo-based: Run version A in a campaign targeting one geography, and version B in a campaign targeting another one, both at the same time (e.g. California vs. Texas).
- Parallel: Create your test version and let it run parallel to the baseline, without splitting users, time, or geography.
Each approach has its pros and cons and limitations.
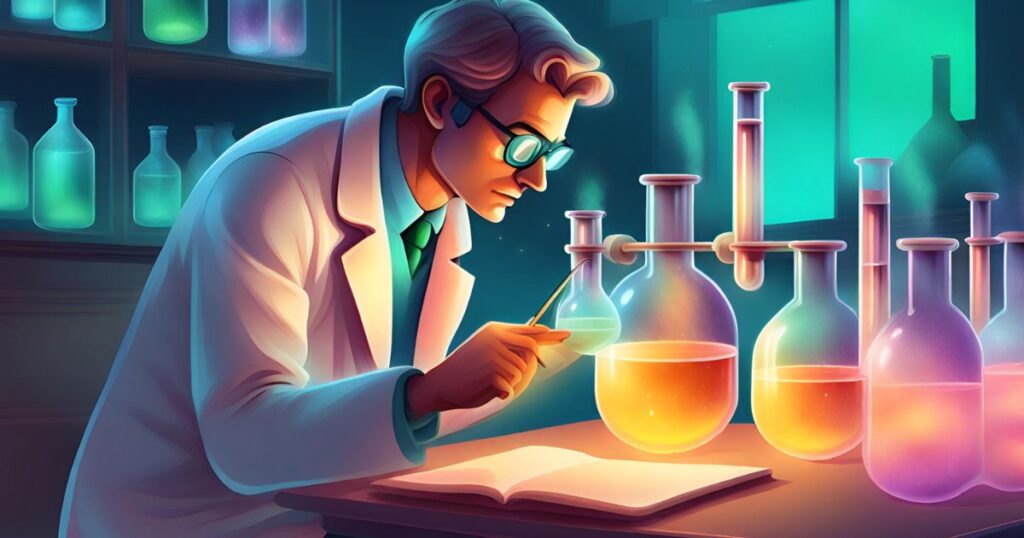
Google Ads Experiments
The ideal approach to A/B testing is a split test in which half of the users will be served version A, and the other half of the users will be served version B of your test. Experiments on Google Ads allow you to do that. I use this approach to A/B test different bid strategies, but you can also use it to test ad variations.
To set up an experiment for a search campaign, follow these steps:
- In Google, go to Experiments > All experiments
- Create new experiment > Custom experiment > Search
- Name your experiment and select the base campaign (control) > Add a suffix to the name of the test campaign
- Click Schedule > Select your experiment goals, experiment budget split and test period
- Go to the test campaign and amend your test variable
Note: If you’re working on an important campaign, it might be wise to test it with a 20% budget split rather than 50%, in case the performance of the test version is lower than expected.
Google experiments are great for campaigns with a significant amount of conversions. The main advantage is controlling all time (weekdays, seasonality) and geographic variables, which allows us to get our results fast and with good statistical validity.
Time-Based A/B Testing
However, experiments aren’t the best option for all tests. For example, for campaigns with too few conversions, a test would take relatively long and the results might not be statistically significant.
For these cases, you might want to consider making your changes to the campaign and then comparing the results ‘before’ and ‘after’.
The advantage of this approach is that there’s no need to split the budget and conversions between two campaigns. However, if the change had a negative impact on the campaign’s performance, it affects everything, and not only 20%-50% percent.
Another downside of this approach is the time-related differences between the two periods. For example, if your ‘before’ period is October, and your ‘after’ period is November (weather, BFCM, holiday season), these two periods are very different in terms of volumes and purchase intent.
Geo-Based A/B Testing
Geo-based split testing is a great way to test changes on a limited part of your audience. For example, to test a new landing page, or even the impact of a display campaign, where direct impact on sales isn’t always visible on Google Ads.
You can then compare the results in the test geography to the overall baseline. Did we a change in sales in California, where we ran the display campaign, vs. Texas or the rest of the US?
The advantage of geo-based vs. time-based A/B testing is that we control the testing period, which is identical for both versions.
The disadvantage is that we need to assume that our test geography is similar to our control geography, where we didn’t make changes or create a new campaign.
Parallel A/B Testing
It’s not always possible, or it doesn’t make sense to split-test.
For example, If you’d like to test audience signals in a PMax campaign, I’d recommend setting up a new asset group with different audience signals and letting it run side by side with the original asset group.
The bidding algorithm should already optimize the budget split between the two asset groups.
If you identify a clear winner, you can pause the other asset group. But make sure to keep an eye not only on CPA and ROAS, but also on volumes. (spend and conversions).
Other cases for parallel testing are ad copy and landing pages, adding or excluding keywords, and different keyword match types (how did adding broad keywords affect your CPA?).
Analyzing Test Results
There are several things to consider when analyzing results from an A/B test:
- Make sure to allow enough time for your changes to kick in. The bidding algorithm needs time to recalibrate, and your campaign should be given the time to collect enough conversions. With too few conversions, metrics fluctuate and are more sensitive to flukes.
- Focus on your main metric for this test. If you wanted to increase conversions within the same budget, did it work? Did the results meet your hypothesis? If not, what might be the reasons?
- Don’t ignore other metrics. For example, in one of my accounts I tested tROAS vs. tCPA strategy. While the tROAS version resulted in improved ROAS and CPA, the search impression share decreased, which resulted in fewer conversions.
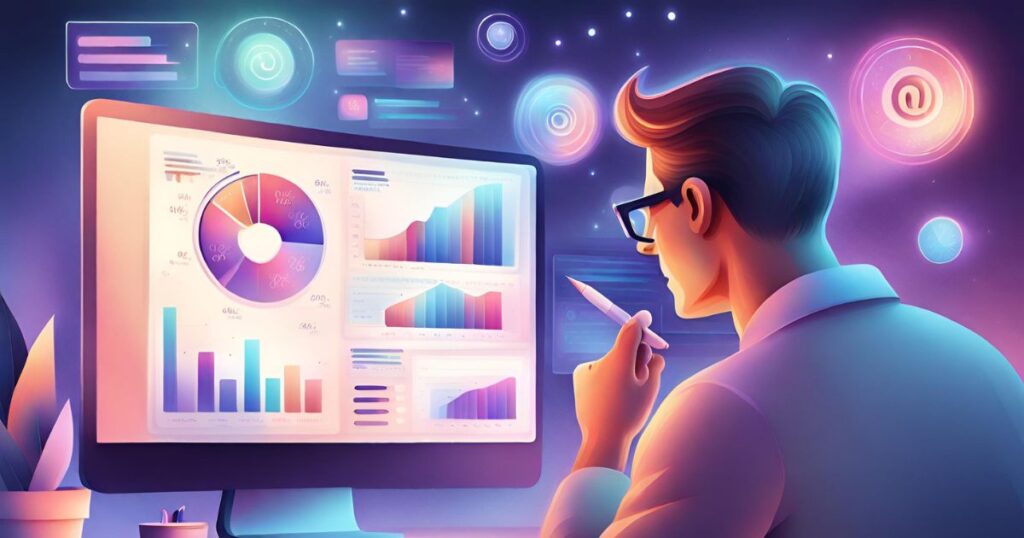
Implementing Results
Once you have clear winners and losers, it’s time to pause the losers and start planning the next test. Maybe a new ad angle? Maybe testing the impact of high-funnel display and YouTube campaigns on brand searches? Or assigning your products to separate PMax campaigns based on margins and performance?
If you tested a certain change on several campaigns already and it brought positive results, you can gradually roll it out to more campaigns. Just make sure to keep your eyes open, in case it doesn’t work for a specific campaign.
The important thing to keep in mind is to never stop testing!
A/B Testing Best Practices
Here are a few best practices for your A/B testing strategy:
- Align your objectives with your account strategy
- If you took on a new account, plan a testing roadmap for the first period
- Identify the most relevant metric when you plan the test
- Refer to the same metric when analyzing the results
- Only change one variable at a time
- Document your tests for future reference and insights
- Test different elements – bid strategies, ad copy, landing pages, campaign structure etc.
- Gather enough data before picking a winner (or a loser)
Conclusion
A/B testing is an essential approach digital marketers use to continuously improve the results of their ad campaigns.
A solid A/B testing strategy can expose what works and what doesn’t in the Google account or in general, and help identify new opportunities for growth.
By running structured A/B testing of bid strategies, campaign structure, and ad variations, Google advertisers can significantly optimize their results over time, and help businesses grow and improve profit margins.
Further Reading
- Facebook Ad Testing: A/B Test To Improve Your Results
- Digital Marketing Insights From Managing Campaigns for 20 Online Shops
- Google Ads vs. Facebook Ads For Ecommerce: Which Advertising Platform Is Better For Your Business?
- Spying On Google Ads: PPC Competitor Analysis
- 5 Performance Max Best Practices To Nail Sales